How to Optimize Customer Analytics to Improve the Post-Purchase Customer Experience
Teresa Wingfield
September 26, 2023
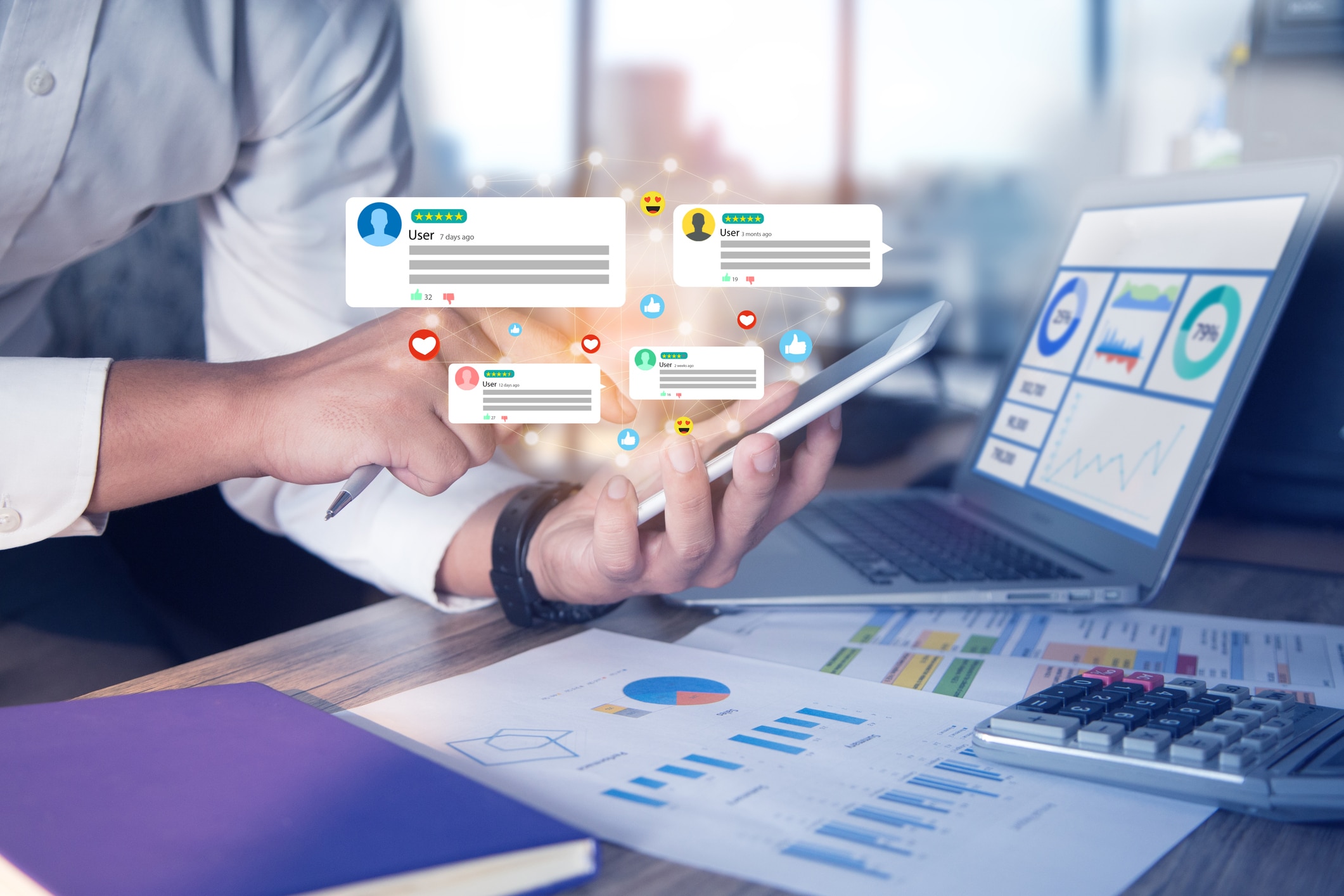
In a recent Martechcube survey, only 18% of retail leaders believe that they could significantly improve the post-purchase customer experience. In contrast, a whopping 80% of consumers feel otherwise. Providing a poor post-purchase customer experience can prevent you from building customer loyalty. Customer analytics can provide valuable insights and data-driven strategies to help you get to know your customers, personalize customer experiences, and improve customer satisfaction.
Over-Reliance on Customer Segmentation
One of the biggest culprits underlying a poor post-purchase customer experience is segmentation. Analytics allows you to segment your customers into similar groups with similar characteristics such as income, gender, age, etc., or behaviors such as purchases, path-to-purchase, and promotional responses.
Marketers use segmentation to help them tailor their campaigns, promotions, and communication to each segment, hoping that these will resonate with customers in the same segment. But do they? Not always. People falling within a segment often have different needs, values, and motivations, and, even if they have the same behaviors, their reasons or motivations for that behavior can be very different.
Insufficient Personalization
By analyzing a customer’s purchase history, browsing behavior, demographics, and other customer activities, you can deliver targeted content, product recommendations, and offers that are more likely to resonate with the customers. More savvy retailers are bringing zero-party data into the personalization mix. Zero-party data is information from customers that they voluntarily and deliberately share with you. The use of zero-party data has risen in popularity after Google announced its intended phase-out of support for third-party tracking cookies in Chrome back in early 2020. Since this time, marketers have realized that zero-party data is more than a replacement strategy for cookie data and now understand that one of the best ways to know what a customer wants is to simply ask the customer.
Predictive Analytics Can’t Always Forecast Churn
There’s no doubt that predictive analytics is a valuable tool that can help you predict customer behavior, such as their likelihood of churning or making a repeat purchase. Insights can assist you in proactively addressing issues and engaging at-risk customers.
On the downside, there are tons of factors that cause predictive analytics to fail to predict customer churn. Insufficient or poor-quality data will impact the accuracy of results for any type of modeling. Predictive models base their predictions on trends in historical data. As such, they might fail to predict that a customer has decided to churn abruptly due to a recent negative experience. This is a big shortfall for predictive accuracy because 76% of shoppers will stop doing business with a company after just one negative experience. In addition, the competitive landscape is constantly evolving, and historical data may not reveal this.
These shortcomings have several implications for users of predictive analytics. It’s important to regularly update predictive analytics models, validate results, and incorporate a variety of data sources, both internal and external. Also, predictive analytics needs to be part of a comprehensive data analytics approach that includes adaptive analytics strategies. For example, analyzing current data from customer support interactions, including call logs, chat transcripts, and email can quickly identify if a customer is experiencing an issue. And keeping track of new social media mentions and conversations can help you spot unhappy customers faster.
Let’s Make CX Easy Together
Customer analytics provide valuable insights to help you know your customers better to help you deliver a more engaging customer experience. But more is needed than traditional segmentation. You’re going to need to focus more on individual customers and engage with them directly to understand their needs. Advanced analytics such as predictive modeling are useful for understanding future customer behavior, but you’ll still need adaptive analytics to identify sudden changes in the customer experience or market dynamics.
According to a recent GigaOm TPC-H Benchmark Test, the Actian Data Platform operational data warehouse is 9x faster and 16x cheaper than alternatives. The Actian Data Platform makes it easy to track, manage, and analyze customer analytics to better identify areas that need improvement and help improve business outcomes. Contact us to start your journey to improving CX.
Subscribe to the Actian Blog
Subscribe to Actian’s blog to get data insights delivered right to you.
- Stay in the know – Get the latest in data analytics pushed directly to your inbox
- Never miss a post – You’ll receive automatic email updates to let you know when new posts are live
- It’s all up to you – Change your delivery preferences to suit your needs