Optimizing Cloud Compute and Data Storage for Data Analytics
Actian Corporation
October 24, 2019
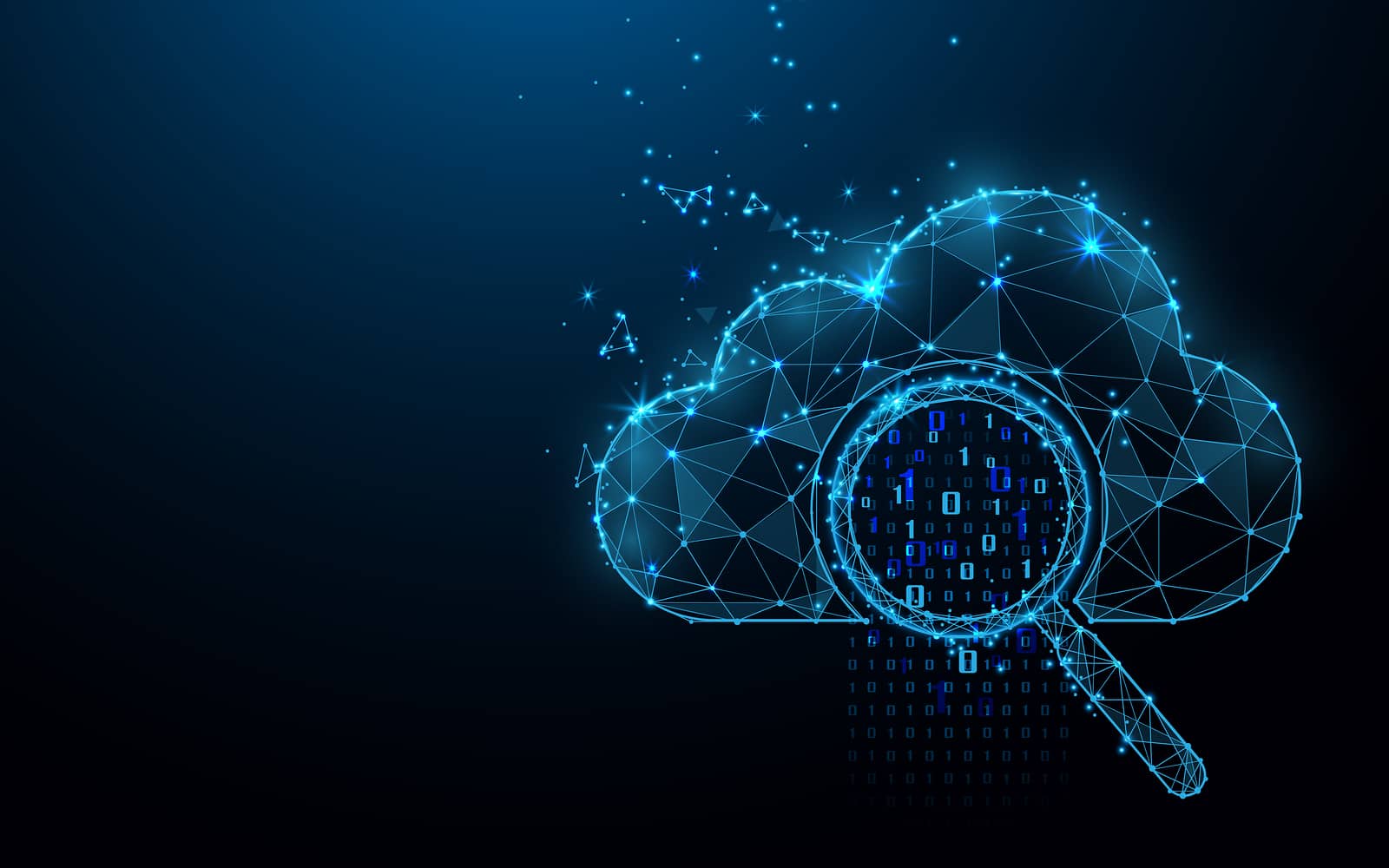
When architecting your data warehouse solution, separating compute and data storage is extremely important for both operational sustainability and economic efficiency. Different things drive the technical needs for each of these, the capacity demands of the organization are different, and the best solution requires optimizing compute and data storage separately.
Storage Capacity is a Function of Time
The amount of data storage that your company needs is directly related to the number of business activities you’re doing. As you conduct business, you generate data – data about your customers, your products, your sales, etc. Over time, the amount of data volume your company has will grow. In busy times, the growth rate may be faster than in slow times, but the volume is always increasing. Taking this growth into account is essential when you are architecting your storage solution because the cost is directly related to data volume.
For on-premises data storage, you will need to acquire capacity in advance, based on projected data storage needs. For cloud-based storage solutions that are billed based on utilization, you will need to project your cost growth over time.
Compute Capacity is Driven by Business Trends
Compute capacity for analytics solutions is only partially influenced by the volume of data you are analyzing. The more significant factor at play is the demand for data consumption – during peak business times, the demand is higher, and during slow times, the demand is lower. Consider the example of black Friday in retail. Business activity spikes, and the demand for analytics about the business activities spike too. A couple of months later, in early January, retail sales slowed, and there was also less demand for analytics. Whether you are talking about retail sales, the launch of a new product/service, or quarter-end financial close, every business has seasonality trends that cause their demand for compute capacity to vary significantly.
For on-premises compute solutions, capacity must be purchased and reserved to accommodate peak performance loads. That means that during slow periods, there is excess capacity. For cloud-based compute solutions where billing is based on utilization, capacity can be scaled up during peak periods and scaled back down during slow periods.
Developing a Hybrid Demand Forecast is Nearly Impossible
The capacity and performance requirements for both compute and data storage environments vary over time based on the activities of the business. The demand curves for each of these solutions look very different from the other, making cost and capacity modeling based on a combined architecture is both difficult and inefficient. Rather than invest the time and resources on-demand and cost forecasting, most companies find it much easier to separate compute and data storage into separate solutions with independent cost models and demand forecasts.
Technology is Changing
Cloud-based technology capabilities are improving and changing at a tremendous pace. When it comes to data analytics and cloud data warehousing, not only is the technology getting better every day, but certain areas are evolving faster than others. For example, the density of cloud-based storage solutions is causing the per-unit cost of data storage to decline in alignment with the deployment of new hardware by cloud service providers. Compute capabilities in the cloud are improving in both capacity/scale with new distributed compute architectures, and in speed/performance with new hardware. While a company may decide to forego a storage upgrade due to the migration costs, leveraging newer compute capabilities may be advantageous.
Separating compute and data storage solutions give companies greater flexibility in upgrading parts of their architecture while leaving other parts alone. When it comes to data analytics, there are a lot of moving parts. Data volumes are increasing. Analytics and compute demands (both performance and capacity) are going up and down with business trends. Developing and executing on an accurate forecast is nearly impossible. All the while, the technology is continuously evolving and the business is demanding better economic performance from IT investments. Companies that are thriving in this environment know that keeping solutions simple and maintaining the highest level of technical flexibility is the key to success. Separating compute and data storage is an essential part of giving you the most options to optimize the data analytics on which your company depends.
Actian Data Platform on Azure provides the flexibility organizations need to optimize the ratio of compute and data storage to meet the performance objectives of the application. Learn more about Actian Data Platform at www.actian.com/data-platform
Learn Modernization Best Practices from Industry Experts and Insiders
If you are thinking about modernizing your enterprise data warehouse, watch our on-demand webinars featuring leading industry analysts and former executives from Teradata and Netezza.
Subscribe to the Actian Blog
Subscribe to Actian’s blog to get data insights delivered right to you.
- Stay in the know – Get the latest in data analytics pushed directly to your inbox
- Never miss a post – You’ll receive automatic email updates to let you know when new posts are live
- It’s all up to you – Change your delivery preferences to suit your needs