GenAI Best Practices for Data Scientists, Engineers, and IT Leaders
Vamshi Ramarapu
November 16, 2023
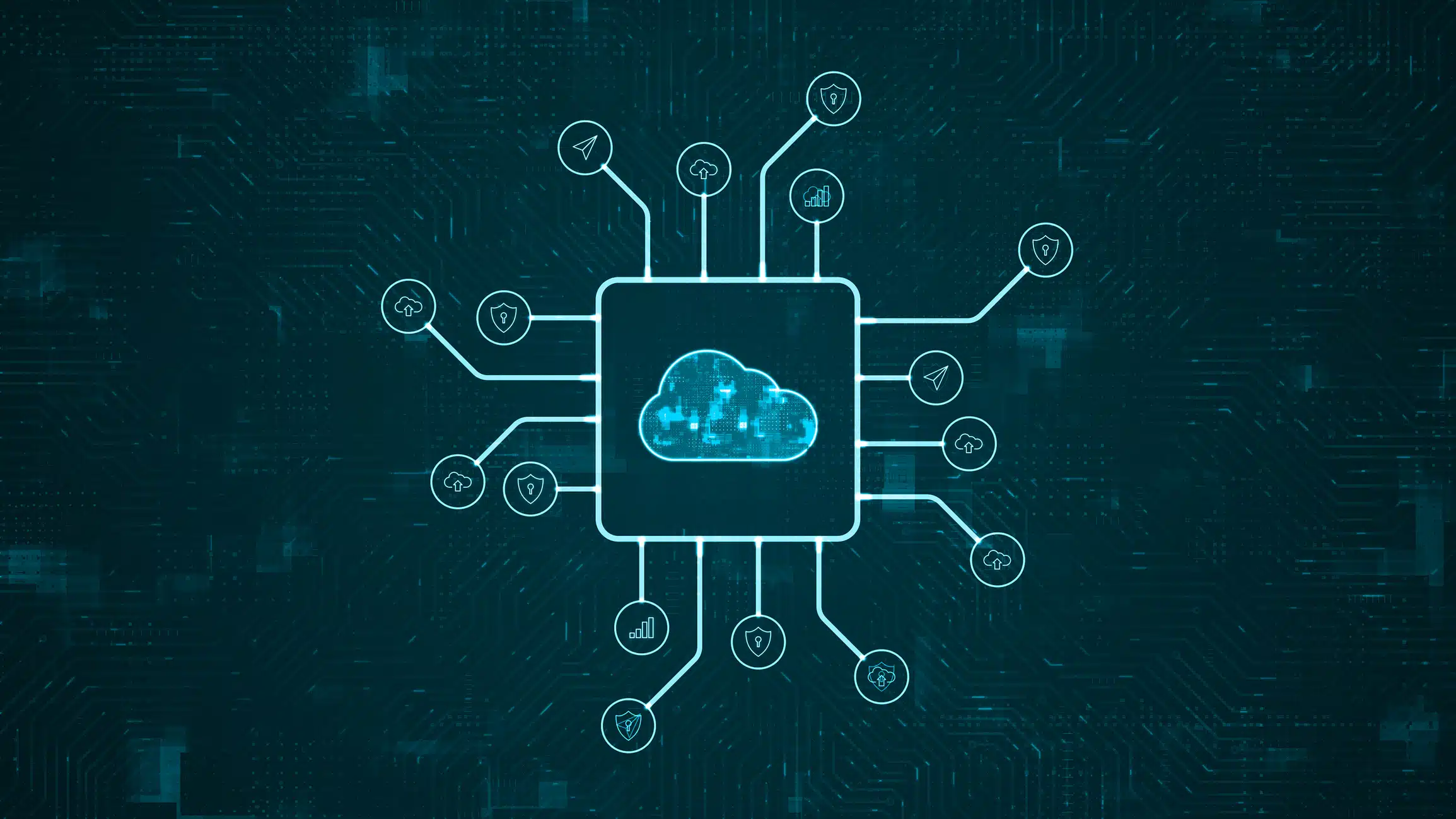
As organizations seek to capitalize on Generative AI (GenAI) capabilities, data scientists, engineers, and IT leaders need to follow best practices and use the right data platform to deliver the most value and achieve desired outcomes. While many best practices are still evolving GenAI is in its infancy.
Granted, with GenAI, the amount of data you need to prepare may be incredibly large, but the same approach you’re now using to prep and integrate data for other use cases, such as advanced analytics or business applications, applies to GenAI. You want to ensure the data you gathered will meet your use case needs for quality, formatting, and completeness.
As TechTarget has correctly noted, “To effectively use Generative AI, businesses must have a good understanding of data management best practices related to data collection, cleansing, labeling, security, and governance.”
Building a Data Foundation for GenAI
GenAI is a type of artificial intelligence that uses neural networks to uncover patterns and structures in data and then produces content such as text, images, audio, and code. If you’ve interacted with a chatbot online that gives human-like responses to questions or used a program such as ChatGPT, then you’ve experienced GenAI.
The potential impact of GenAI is huge. Gartner sees it becoming a general-purpose technology with an impact similar to that of the steam engine, electricity, and the internet.
Like other use cases, GenAI requires data—potentially lots and lots of data—and more. That “more” includes the ability to support different data formats in addition to managing and storing data in a way that makes it easily searchable. You’ll need a scalable platform capable of handling the massive data volumes typically associated with GenAI.
Data Accuracy is a Must
Data preparation and data quality are essential for GenAI, just like they are for data-driven business processes and analytics. As noted in eWeek, “The quality of your data outcomes with Generative AI technology is dependent on the quality of the data you use.
Managing data is already emerging as a challenge for GenAI. According to McKinsey, 72% of organizations say managing data is a top challenge preventing them from scaling AI use cases. As McKinsey also notes, “If your data isn’t ready for Generative AI, your business isn’t ready for Generative AI.”
While GenAI use cases differ from traditional analytics use cases in terms of desired outcomes and applications, they all share something in common—the need for data quality and modern integration capabilities. GenAI requires accurate, trustworthy data to deliver results, which is no different from business intelligence (BI) or advanced analytics.
That means you need to ensure your data does not have missing elements, is properly structured, and has been cleansed. The prepped data can then be utilized for training and testing GenAI models and gives you a good understanding of the relationships between all your data sets.
You may want to integrate external data with your in-house data for GenAI projects. The unified data can be used to train models to query your data store for GenAI applications. That’s why it’s important to use a modern data platform that offers scalability, can easily build pipelines to data sources, and offers integration and data quality capabilities.
Removing Barriers to GenAI
What I’m hearing from our Actian partners is that organizations interested in implementing GenAI use cases are leaning toward using natural language processing for queries. Instead of having to write in SQL to query their databases, organizations often prefer to use natural language. One benefit is that you can also use natural language for visualizing data. Likewise, you can utilize natural language for log monitoring and to perform other activities that previously required advanced skills or SQL programming capabilities.
Until recently, and even today in some cases, data scientists would create a lot of data pipelines to ingest data from current, new, and emerging sources. They would prep the data, create different views of their data, and analyze it for insights. GenAI is different. It’s primarily about using natural language processing to train large language models in conjunction with your data.
Organizations still want to build pipelines, but with a platform like the Actian Data Platform, it doesn’t require a data scientist or advanced IT skills. Business analysts can create pipelines with little to no reliance on IT, making it easier than ever to pull together all the data needed for GenAI.
With recent capability enhancements to our Actian Data Platform, we’ve enabled low code, no code, and pro code integration options. This makes the platform more applicable to engage more business users and perform more use cases, including those involving GenAI. These integration options reduce the time spent on data prep, allowing data analysts and others to integrate and orchestrate data movement and pipelines to get the data they need quickly.
A best practice for any use case is to be able to access the required data, no matter where it’s located. For modern businesses, this means you need the ability to explore data across the cloud and on-premises, which requires a hybrid platform that connects and manages data from any environment, for any use case.
Expanding Our Product Roadmap for GenAI
Our conversations with customers have revealed that they are excited about GenAI and its potential solutions and capabilities, yet they’re not quite ready to implement GenAI technologies. They’re focused on getting their data properly organized so it’ll be ready once they decide which use cases and GenAI technologies are best suited for their business needs.
Customers are telling us that they want solid use cases that utilize the strength of GenAI before moving forward with it. At Actian, we’re helping by collaborating with customers and partners to identify the right use cases and the most optimal solutions to enable companies to be successful. We’re also helping customers ensure they’re following best practices for data management so they will have the groundwork in place once they are ready to move forward.
In the meantime, we are encouraging customers to take advantage of the strengths of the Actian Data Platform, such as our enhanced capabilities for integration as a service, data quality, and support for database as a service. This gives customers the benefit of getting their data in good shape for AI uses and applications.
In addition, as we look at our product roadmap, we are adding GenAI capabilities to our product portfolio. For example, we’re currently working to integrate our platform with TensorFlow, which is an open-source machine learning software platform that can complement GenAI. We are also exploring how our data storage capabilities can be utilized alongside TensorFlow to ensure storage is optimized for GenAI use cases.
Go From Trusted Data to GenAI Use Cases
As we talk with customers, partners, and analysts, and participate in industry events, we’ve observed that organizations certainly want to learn more about GenAI and understand its implications and applications. It’s now broadly accepted that AI and GenAI are going to be critical for businesses. Even if the picture of exactly how GenAI will be beneficial is still a bit hazy, the awareness and enthusiasm are real.
We’re excited to see the types of GenAI applications that will emerge and the many use cases our customers will want to accomplish. Right now, organizations need to ensure they have a scalable data platform that can handle the required data volumes and have data management practices in place to ensure quality, trustworthy data to deliver desired outcomes.
The Actian Data Platform supports the rise of advanced use cases such as Generative AI by automating time-consuming data preparation tasks. You can dramatically cut time aggregating data, handling missing values, and standardizing data from various sources. The platform’s ability to enable AI-ready data gives you the confidence to train AI models effectively and explore new opportunities to meet your current and future needs. The Actian Data Platform can give you complete confidence in your data for GenAI projects.
Additional Resources:
Subscribe to the Actian Blog
Subscribe to Actian’s blog to get data insights delivered right to you.
- Stay in the know – Get the latest in data analytics pushed directly to your inbox.
- Never miss a post – You’ll receive automatic email updates to let you know when new posts are live.
- It’s all up to you – Change your delivery preferences to suit your needs.