What is a Modern Data Platform?
Actian Corporation
October 9, 2020
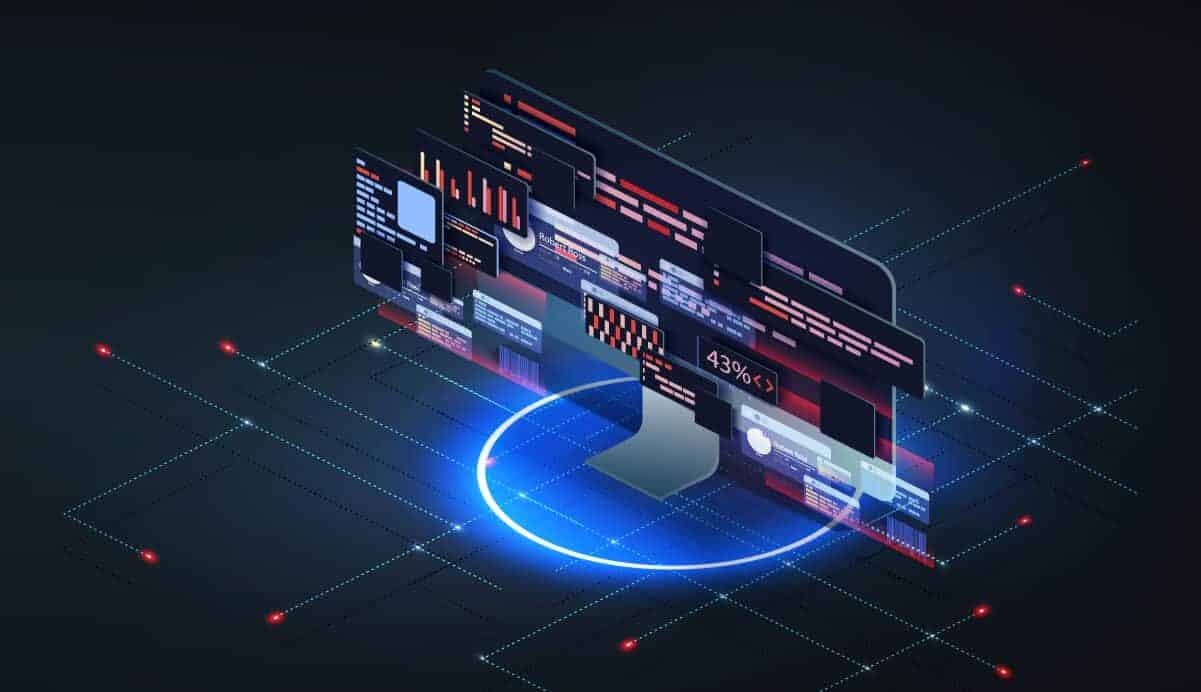
What is a modern data platform? When we ask this question, we get two different groups of answers. The first group is around the technology needed for data processing in today’s business environment. In this group, the answers mention things like cloud services, containers, and on-prem. The second set of answers involves sources of data, types of data, and the management of that data. This group is more focused on using the data that is collected, but the fact is that both groups need to work together to provide a modern data platform.
Modern Data Platform Technology
The modern data platform has to deal with three different aspects of business data.
1. Volume – This is perhaps the easiest aspect to deal with in storage systems that utilize cloud technologies. Products like Actian can be delivered on hybrid systems that include private and public cloud service providers, including Amazon Web Services, Microsoft Azure, and Google Cloud. These platforms all offer the capability to grow to support increasing volumes of data and, in many cases, offer the ability to maintain that data for long periods.
2. The Variety of Data Types and Input Sources – Most people have multiple digital devices and interact with multiple executable sources. A single individual may use a mobile phone, a tablet, and a computer to interact with a given business. More than direct interaction, inputs may come from social media applications, and mobile phone apps in the form of structured or semi-structured data. One of the keys to a successful modern data platform is the utilization and dependency on standard protocols rather than some proprietary connection.
3. The Velocity of Data Accrual – Because data arrives from a variety of sources, it tends to arrive quickly as well. The consolidation of disparate data sources is another key to the modern data platform. It is difficult at best to bring together all of the data into a single repository or format, so virtual unity through data management and operations is necessary. One way to accomplish the virtual consolidation is through adaptive indexing and metadata use. Replacing or augmenting traditional taxonomies with faceting classifications enables the data to be searched and organized in multiple ways. This provides the use of different analytics and allows organizations to understand the data.
Beyond the technology is the need for a business to understand the data that they have, what additional data they might need, and how all of that data can be used.
Data Usage
The goal for any organization is data-driven insights into business operations and business needs. Those insights are only achievable based on data pipelines that are cleansing, formatting, and organizing the data. The data pipeline is fed from the business data operations. Data operations are, in turn, fed by the raw data collection and our data repositories. That data should be accessed via standard protocols for things like workflows, data quality measures, and data governance.
The insights derived from the process need, in turn, to be distributed to the appropriate consumers in order to provide value. That distribution can be in the form of some combination of reports, service layer APIs for automated action, and mobile device alerts. The delivery of value based on the processes of a modern data platform needs to align with the needs of the users of the processed data. Dashboards and reports on things like financial and operational performance can be actionable data products. That means that reports and dashboards are important, but so also is information in a form that takes a step beyond reporting. For example, linking regulatory or previously stored data with current data would be useful when processing invoice data in a financial system.
From a business perspective, the types of data are an important aspect of the data platform. Customer data was ranked in a recent survey as the most important data in a data warehouse. Businesses want to turn prospects into customers, customers into loyal customers, and loyal customers into product advocates. In order to do that, good customer data collection and processing is necessary in order to build aggregated customer data to identifies customer groups and segments.
Best practices for managing customer data include:
- Each customer has a single source record, no matter how many data sources there are.
- Access to customer data should have standard governance for storage, retrieval, and usage.
- Data that is not relevant to the business relationship should not be stored or processed.
- Customer privacy needs to be protected.
The modern data platform needs to manage multiple types of data. In the survey, transactional data is listed as the second most important type of data. Transactional data is often the result of customer interactions, such as product purchases, product returns, payments, subscriptions to newsletters and other recurring information, and where applicable, donations. These types of data often have legal significance in addition to business significance. Other common types of data include operational data, contact center data, marketing data, and reservations.
Once a business has decided on what data is important to collect, then the data platform needs to be deployed and monitored. Data needs to be processed in a timely manner, appropriately governed, and protected. The data storage needs to be managed as well as data access and usage.
Learn more about Actian – hybrid data warehouse platform here.
Subscribe to the Actian Blog
Subscribe to Actian’s blog to get data insights delivered right to you.
- Stay in the know – Get the latest in data analytics pushed directly to your inbox.
- Never miss a post – You’ll receive automatic email updates to let you know when new posts are live.
- It’s all up to you – Change your delivery preferences to suit your needs.