Data immaturity can put you out of business
Merriam-Webster defines maturity as (1) the quality or state of being mature or (2) termination of the period that an obligation has to run. While the first definition is of little use, the second forms the basis for this discussion on maturity. Data Management Maturity (DMM) guides your obligations for:
- The ways data are handled and used.
- Governance and regulatory responsibilities.
- Security, recovery, archival and backup requirements.
- Ease of use by staff and customers.
- Cost of data management and storage.
In 2017, Harvard Business Review released a study revealing that only 3% of companies’ data met quality standards, and even worse, 47% of newly created data records had at least one critical error. An MIT Sloan Management Review article argues that 15-25% of your revenue can be lost due to insufficient data, which led Bill Gates to say, “How you gather, manage, and use information determines whether you win or lose.”
What is Data Management Maturity ?
Data maturity relates to how you measure yourself against a recognized standard of what it should be, the processes used to manage and access data, the tools employed, and the data used in decision-making.
According to ISACA, the gold standard for process improvement, CMMI’s data management maturity model is:
“a process improvement and capability maturity framework for the management of an organization’s data assets and related activities. It contains best practices for establishing, building, sustaining, and optimizing effective data management across the data lifecycle, from creation through delivery, maintenance, and archiving.”
CMMI cautions that the proposed 5 levels below should be used as a guide and that organizations apply each level according to their unique situation.
The five levels of the CMMI Data Management Maturity model guide your performance against risk, capability, and usage are:
- Performed processes are performed ad hoc, primarily at the project level. Process discipline is primarily reactive, fixing data issues rather than improving quality processes. Data is considered only from the project, application, or immediate work tasks and not as a strategic resource. Data management is not a board-level initiative or topic.
- Managed processes are now planned and executed within policy guidelines. While there is awareness of the importance of treating data as a critical asset, the skills or tools are still inadequate from an organizational perspective. Senior leadership has begun to take data management seriously and is guided by assessments from consultants or industry standards.
- Defined sets of standard processes are now helping to provide a consistent quality of data to help perform business tasks, meet strategic visions or maintain regulatory compliance. Management and governance oversight has been introduced along with monitoring, alerting and feedback loops. Data inconsistencies have the resources, tools, and funding to be addressed for critical datasets.
- Measured managed and process metrics are judged against agreed variances. Data is treated as a source of competitive advantage or as an asset in performing daily tasks. Everyone is using data as a source of information and is concerned about its accuracy and timeliness to perform their work safely and securely. Applications are written to capture data issues that are resolved as quickly as possible to avoid reputational damage or regulatory fines.
- Optimized process performance is continually improved through incremental and innovative improvements driven by feedback obtained via automated tools, peers, industry practices, competitors, and customers. Data is regarded as the critical asset, other than skilled resources, for survival in a volatile economy.
As maturity and awareness improve, creating a Master Data Management (MDM) strategy and model specific to your organization emerges. MDM helps ensure that your data becomes information that can integrate the way your company works, makes decisions, influences customers, achieves its goals and continues to remain competitive, secure, compliant, sustainable and relevant. A data management framework will be composed of both the MDM and the DMM.
Model of DMM CMMI
ISACA and CMMI merged in 2016. Between them, they have articulated the model for how data can best be obtained, used, kept, and deleted, which is now the gold standard, as seen above. Their model encompasses six categories, each with several processes or practices for Data management strategy, data governance, data quality, data operations, and platform architecture. The DMM model highlights that your organization must evaluate and set each category and its functions. Data need, and usage is unique, and the model can only act as a guide by creating an agreed framework for behavior, tools, and culture for data and information.
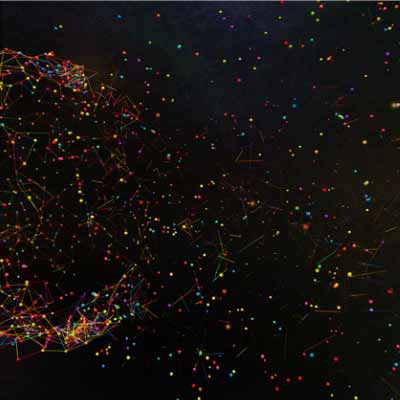
Making the data management model your model
Consider the intent and breadth of CMMI’s DMM against your enterprise data management model and processes. Data is your most crucial non-people asset; therefore, your DMM must consider all of these concepts to be of any value:
- Data management strategy.
- Enterprise data management processes.
- Communications to staff, customers, and partners.
- Business case and funding.
- Data governance and a business glossary of terms.
- Metadata management tables and templates.
- Data quality strategy.
- Data profiling and cleansing.
- Data and data management assessment.
- Data requirements.
- Data governance and control.
- Data platform, architecture and configuration management.
- Data reporting.
- Data management backup, archival, and recovery.
- Data management analysis.
- Risk management.
Those organizations that take the time to create a viable, flexible and user-friendly data management model will be the ones to benefit from a digital business while keeping the costs of data access and use under control.
Benefits of a model and maturity
The DIKW pyramid states that data becomes Information used in daily tasks. Data then becomes knowledge underpinning decisions and aids in the wisdom of solving problems or fulfilling requests. To achieve wisdom data must be:
- Trusted.
- Enhance the quality and timeliness of decisions.
- Reduce risk.
- Help to manage costs.
- Maintain compliance.
- Sustain competitiveness.
- Increase productivity.
- Ensure agility for product introduction and maintenance.
How to start
The Capability Maturity Model has undergone many iterations since it was first created in 1991. This model aids leaders by asking them to review what happens if they do not manage data and how do they know that what they are doing is acceptable?
Organizations fail in their attempt when they strive to use the DMM in its strictest sense without applying it to their circumstances
The Data Maturity Model, like many other Capability Maturity Models, were never meant to be used as-is. Instead, best practice is to ensure the wording of each level, including the level names or and goals, have relevance to your teams and management. For instance, one client changed their model names to be:
- We don’t do this well.
- We are beginning to understand.
- We now have a standard practice.
- We are pretty good at this.
- We are really good at this.
The teams then created wording for each level with outcome key result indicators to act as guardrail guides and warnings when they were not on track to improve how they were managing data. Start a pilot, get staff involved, make the model apply to each area of your company, measure performance or customer impact. Regularly review practices with auditors or via software tools to assess the state of your data and the maturity of your methods.
The last point is quite essential. With the accumulation of data, the ease of introducing data from a wrong source can cause you to incur fines, have reputational damage, lose staff and customer trust, or put you out of business. You will require software, be it tools for monitoring and alerting of issues, your application’s internal data check or cloud products, to help you maintain Data Management Maturity. Use news stories to motivate you to act so that what competitors have done incorrectly does not happen to you. Remember that data management and maturity is the role of everyone!
DevOps, agile or ITSM practices such as value stream mapping can assist in the creation of visual models of data flow and use within your organization. These practices highlight gaps, redundancy, manual processes, or risks, facilitating iterative improvement tasks to encourage trustworthy data management and maturity.
A basic but scalable Data Management Maturity model must encompass the enterprise and treat each domain as a discrete part of the model. Therefore, an overall governance team would include:
- Sponsorship.
- Skill review and training.
- Business case and funding. Collaboration and communication.
- Data culture, especially around security.
- Enforcement of business value.
- Technology use such as cloud, data warehouse and data lakes.
- Data Management Maturity assessment and survey against capabilities, skills, and tools.
Gartner suggests that you take 12 actions to create a Data Governance Maturity Model, which your MDM maturity model will support. The Gartner data governance maturity model includes KPIs, roles, data governance guidelines, tools to consider and how to judge business value and outcomes. It is a flexible alternative to ISACA CMMi.
Data management lifecycle
Data stewardship is the practice of defining and managing data, documenting its use in catalogues, creating policies and rules, cleansing data, monitoring that data introduction and use is as required, de-duplication, access, archival and recovery. Data stewardship underpins Enterprise Data Management (EDM) and MDM architecture, governance, and quality. EDM and MDM concepts will evolve into your centers of excellence by business domain, ensuring sound and safe data management.
Summary of Data Management Maturity
Maturity is unique to every business. It is not about hitting a target or a level. Instead, Data Management Maturity should lead towards improvements in capabilities and tools in data usage. As you continue to improve, the need for compliance is recognized and becomes part of usual business tasks and roles. Data Management Maturity must be iterative and will never have an end date as conditions of data use change in this uncertain and volatile economy. Begin with what data is critical to control today, and via agile practices, benefit from a minimum viable set of data approaches to start your maturity improvement journey.
The high-level steps are:
- Perform a comprehensive assessment of the state and use of your data, processes and applications that use data, where and for what reason it is stored and regulatory rules that you must adhere to.
- Decide on a plan that iteratively fills the gaps and improves the use and cost of data.
- Benefit from industry standards and stories.
- Document what works and what does not.
- Create levels of maturity that make sense to you.
- Ascertain the cost of bad data and introduce metrics to avoid poor practices and culture.
Finally, ensure your staff are not wasting time and effort because of bad data. Look at this graphic from Forbes, which states that many companies’ actions are on cleansing and organizing data. Software tools and strong, but flexible rules, will help ensure that you avoid this waste and, instead, concentrate on transforming data into valuable information.
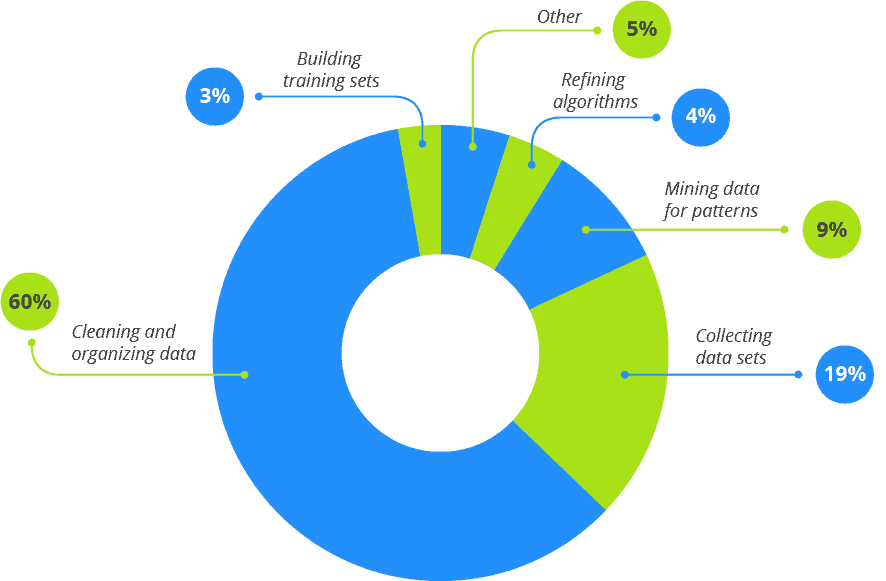