Cluster Analysis: What it is and How to Do One
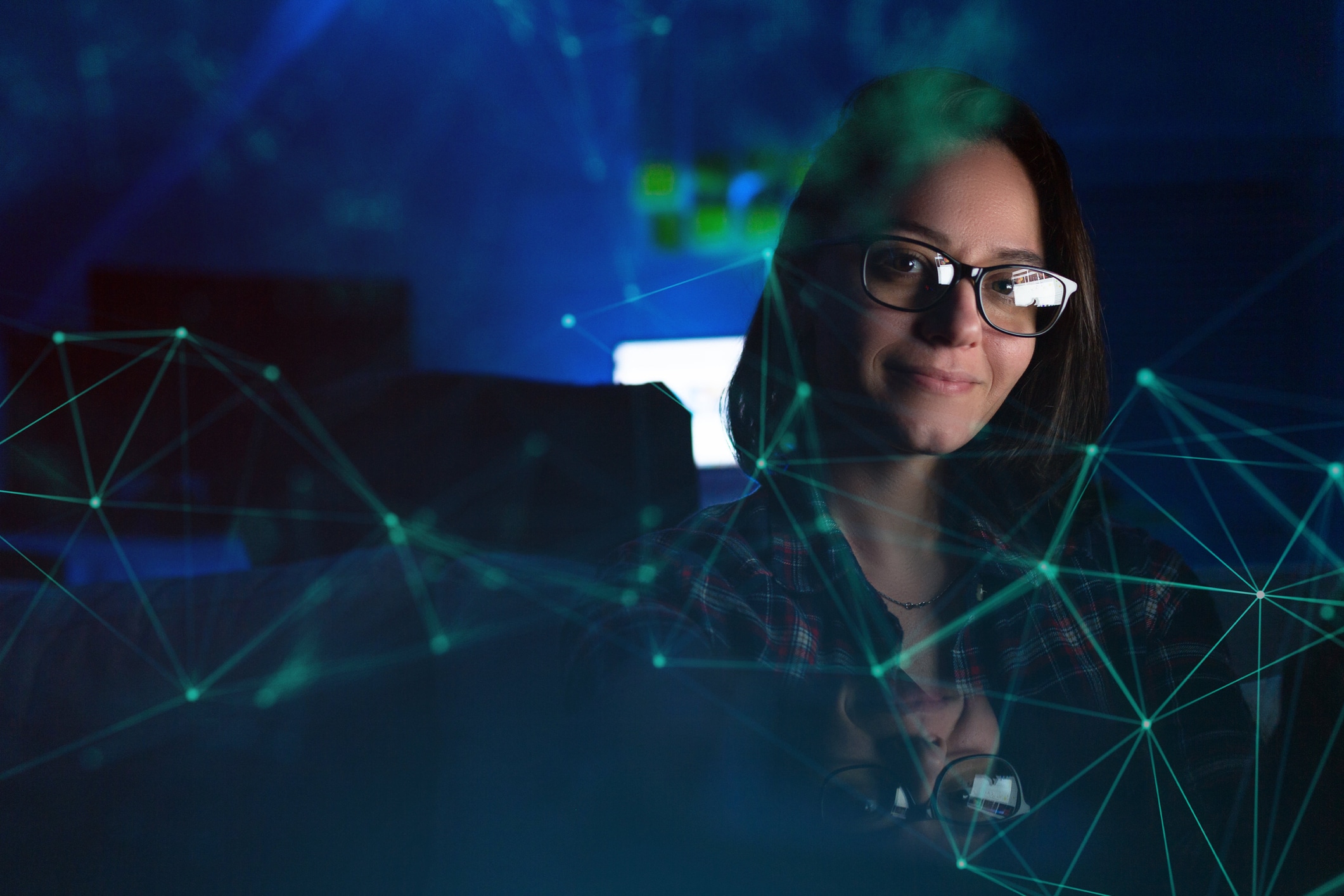
Understanding and making sense of large datasets can be challenging, but identifying patterns and relationships within the data is key to uncovering valuable insights. Cluster analysis helps organizations categorize complex information, enabling better decision-making and strategic planning.
Let’s explore some key terms and concepts related to cluster analysis, helping you confidently navigate its methodologies and applications.
What is Cluster Analysis?
From the viewpoint of Actian, cluster analysis represents a powerful methodology that enables organizations to unlock valuable insights and drive confident decision-making. It is a robust statistical technique that allows businesses to identify meaningful patterns, segment their data, and uncover hidden relationships within complex datasets.
By leveraging cluster analysis, organizations can gain actionable insights, optimize operations, and enhance decision-making. Whether used to segment customers, detect anomalies, improve medical diagnoses, or analyze social networks, cluster analysis is a powerful tool for transforming raw data into meaningful intelligence.
How is Cluster Analysis Used?
Cluster analysis is widely used across various industries to derive meaningful insights from data. Some common applications include:
- Market segmentation: Businesses use cluster analysis to group customers based on purchasing behavior, demographics, or preferences. This enables targeted marketing strategies and personalized customer experiences.
- Anomaly detection: Organizations utilize clustering techniques to identify outliers in financial transactions, cybersecurity threats, or fraudulent activities, helping prevent risks and losses.
- Medical research and diagnosis: Healthcare professionals use cluster analysis to classify diseases, identify patient subgroups with similar symptoms, and enhance personalized treatment plans.
- Image and pattern recognition: In artificial intelligence and computer vision, clustering helps in identifying patterns within images, speech recognition, and object detection.
- Social network analysis: Cluster analysis is employed to detect communities and relationships within social media and networking platforms, aiding in trend analysis and influence mapping.
- Supply chain optimization: Businesses use clustering to segment suppliers, optimize logistics, and enhance demand forecasting, leading to more efficient supply chain management.
These applications showcase the versatility of cluster analysis in helping organizations make data-driven decisions, improve operational efficiency, and gain a competitive advantage.
Methods of Cluster Analysis
There are several methods used in cluster analysis, each with its unique approach to identifying patterns within data. Below are some of the most widely used techniques:
- K-Means clustering: A centroid-based method that partitions data into a predefined number of clusters. Each cluster is represented by a central point (centroid), and data points are assigned to the nearest centroid based on distance metrics. K-Means is efficient for large datasets but requires specifying the number of clusters in advance.
- DBSCAN (Density-Based Spatial Clustering of Applications with Noise): A density-based clustering algorithm that groups together points that are closely packed while marking points in low-density regions as noise. DBSCAN does not require specifying the number of clusters and is effective in identifying clusters of varying shapes and sizes.
- Spectral clustering: A graph-based method that leverages eigenvalues of similarity matrices to perform dimensionality reduction before clustering. It is particularly useful for identifying complex cluster structures and is effective in scenarios where other methods struggle with non-convex shapes.
- Hierarchical clustering: A tree-based approach that builds a hierarchy of clusters either by starting with all data points as individual clusters and merging them (agglomerative) or by starting with a single cluster and splitting it into smaller ones (divisive). This method produces a dendrogram, which can be used to determine the optimal number of clusters.
Each of these methods has strengths and is suited for different types of data and analytical needs. Choosing the right clustering approach depends on factors such as dataset size, cluster shapes, and the desired level of interpretability.
The Advantages of Actian’s Cluster Analysis Solutions
At Actian, we understand the critical role of cluster analysis in extracting actionable knowledge from data. Our solutions provide organizations with the tools and expertise to confidently navigate the intricate landscape of their data, revealing clusters that capture inherent similarities and dissimilarities.
Our cluster analysis solutions offer the following benefits:
Precision and Efficiency
Actian’s cluster analysis solutions offer advanced algorithms and techniques that ensure accuracy, efficiency, and scalability. Our algorithms employ state-of-the-art distance or similarity metrics to capture the nuances of data relationships, allowing for precise and meaningful clustering outcomes.
Confidence in Decision-Making
Confidence is at the core of Actian’s cluster analysis offerings. We work closely with our clients to understand their specific needs, ensuring that the process is aligned with their goals and objectives. Our expert data scientists and analysts guide organizations through every step of the analysis, providing expertise and assurance throughout the journey.
Gaining Actionable Insights
With Actian’s cluster analysis solutions, organizations gain actionable insights into their data. We help businesses identify distinct groups, segments, or patterns within their datasets, enabling them to make informed decisions and tailor strategies based on the characteristics of each cluster. This empowers organizations to optimize their operations, enhance customer targeting, and drive business growth with confidence.
Confidence in Decision-Making
Data security and privacy are paramount considerations in cluster analysis, and Actian takes these concerns seriously. We employ robust data protection measures, including encryption, access controls, and anonymization techniques, to safeguard sensitive information throughout the analysis process. Organizations can trust that their data is handled with the utmost care and security, reinforcing confidence in the cluster analysis outcomes.
Ensuring Data Security and Privacy
Actian’s solutions go beyond just the analysis itself. We provide comprehensive support and consultation to help organizations interpret and leverage the insights derived from cluster analysis effectively. Our visualization tools and reporting capabilities facilitate clear and concise communication of the results, enabling stakeholders to grasp the key findings and confidently take action. Through our comprehensive approach, Actian ensures organizations maximize insights for confident, data-driven decisions.
Partner with Actian for Cluster Analysis Solutions
As an established data company, Actian’s cluster analysis solutions empower organizations to unlock the full potential of their data with unwavering confidence. With advanced algorithms, expert guidance, and a commitment to data security, Actian enables businesses to identify meaningful patterns, uncover hidden relationships, and drive informed decision-making. By leveraging the analysis, organizations can gain a competitive edge, optimize their strategies, and achieve their business objectives with confidence in their data-driven insights. Start your tour of the Actian Zeenea Data Intelligence Platform today.