Quality Data, Reliable AI: Introducing Actian Data Observability
Emma McGrattan
May 12, 2025
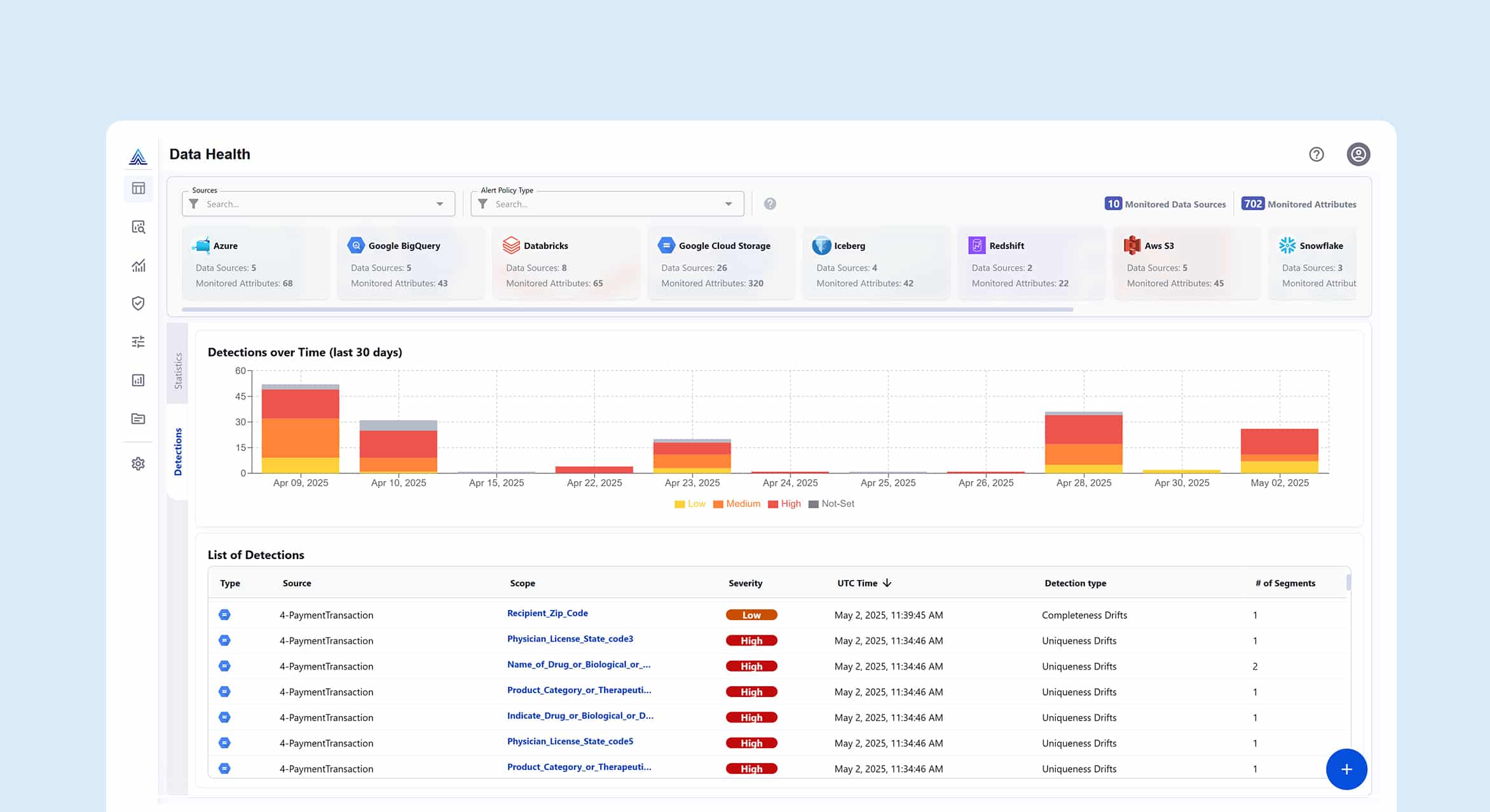
Summary
This blog introduces Actian’s Data Observability platform—a proactive, AI-powered solution designed to ensure data reliability, reduce cloud costs, and support trustworthy AI by monitoring 100% of data pipelines in real-time.
- Proactive AI-powered monitoring prevents data issues: ML-driven anomaly detection identifies schema drift, outliers, and freshness problems early in the pipeline—before they impact downstream systems.
- Predictable costs with full data coverage: Unlike sampling-based tools, Actian processes every data record on an isolated compute layer, delivering no-cost surge assurance and avoiding cloud bill spikes.
- Flexible, open architecture for modern data stacks: Supports Apache Iceberg and integrates across data lakes, lakehouses, and warehouses without vendor lock-in or performance degradation on production systems.
The Real Cost of Reactive Data Quality
Gartner® estimates that “By 2026, 50% of enterprises implementing distributed data architectures will have adopted data observability tools to improve visibility over the state of the data landscape, up from less than 20% in 2024”. But data observability goes beyond monitoring—it’s a strategic enabler for building trust in data while controlling the rising data quality costs across the enterprise.
Today’s enterprise data stack is a patchwork of old and new technologies—complex, fragmented, and hard to manage. As data flows from ingestion to storage, transformation, and consumption, the risk of failure multiplies. Traditional methods can’t keep up anymore.
- Data teams lose up to 40% of their time fighting fires instead of focusing on strategic value.
- Cloud spend continues to surge, driven by inefficient and reactive approaches to data quality.
- AI investments fall short when models are built on unreliable or incomplete data.
- Compliance risks grow as organizations lack the visibility needed to trace and trust their data.
Today’s data quality approaches are stuck in the past:
1. The Legacy Problem
Traditional data quality methods have led to a perfect storm of inefficiency and blind spots. As data volumes scale, organizations struggle with manual rule creation, forcing engineers to build and maintain thousands of quality checks across fragmented systems. The result? A labor-intensive process that relies on selective sampling, leaving critical data quality issues undetected. At the same time, monitoring remains focused on infrastructure metrics—like CPU and memory—rather than the integrity of the data itself.
The result is fragmented visibility, where issues in one system can’t be connected to problems elsewhere—making root cause analysis nearly impossible. Data teams are stuck in a reactive loop, chasing downstream failures instead of preventing them at the source. This constant firefighting erodes productivity and, more critically, trust in the data that underpins key business decisions.
- Manual, rule-based checks don’t scale—leaving most datasets unmonitored.
- Sampling to cut costs introduces blind spots that put critical decisions at risk.
- Monitoring infrastructure alone ignores what matters most: the data itself.
- Disconnected monitoring tools prevent teams from seeing the full picture across pipelines.
2. The Hidden Budget Drain
The move to cloud data infrastructure was meant to optimize costs—but traditional observability approaches have delivered the opposite. As teams expand monitoring across their data stack, compute-intensive queries drive unpredictable cost spikes on production systems. With limited cost transparency, it’s nearly impossible to trace expenses or plan budgets effectively. As data scales, so do the costs—fast. Enterprises face a difficult choice: reduce monitoring and risk undetected issues, or maintain coverage and justify escalating cloud spend to finance leaders. This cost unpredictability is now a key barrier to adopting enterprise-grade data observability.
- Inefficient processing drives excessive compute and storage costs.
- Limited cost transparency makes optimization and budgeting a challenge.
- Rising data volumes magnify costs, making scalability a growing concern.
3. The Architecture Bottleneck
Most data observability solutions create architectural handcuffs that severely limit an organization’s technical flexibility and scalability. These solutions are typically designed as tightly integrated components that become deeply embedded within specific cloud platforms or data technologies, forcing organizations into long-term vendor commitments and limiting future innovation options.
When quality checks are executed directly on production systems, they compete for critical resources with core business operations, often causing significant performance degradation during peak periods—precisely when reliability matters most. The architectural limitations force data teams to develop complex, custom engineering workarounds to maintain performance, creating technical debt and consuming valuable engineering resources.
- Tightly coupled solutions that lock you into specific platforms.
- Performance degradation when running checks on production systems.
- Inefficient resource utilization requiring custom engineering.
Actian Brings a Fresh Approach to Data Reliability
Actian Data Observability represents a fundamental shift from reactive firefighting to proactive data reliability. Here’s how we’re different:
1. Proactive, Not Reactive
TRADITIONAL WAY: Discovering data quality issues after they’ve impacted business decisions.
ACTIAN WAY: AI-powered anomaly detection that catches issues early in the pipeline using ML-driven insights.
2. Predictable Cloud Economics
TRADITIONAL WAY: Unpredictable cloud bills that surge with data volume.
ACTIAN WAY: No-cost-surge guarantee with efficient architecture that optimizes resource consumption.
3. Complete Coverage, No Sampling
TRADITIONAL WAY: Sampling data to save costs, creating critical blind spots.
ACTIAN WAY: 100% data coverage without compromise through intelligent processing.
4. Architectural Freedom
TRADITIONAL WAY: Vendor lock-in with limited integration options.
ACTIAN WAY: Open architecture with native Apache Iceberg support and seamless integration across modern data stacks.
Real-World Impact
Use Case 1: Data Pipeline Efficiency With “Shift-Left”
Transform your data operations by catching issues at the source:
- Implement comprehensive DQ checks at ingestion, transformation, and source stages.
- Integrate with CI/CD workflows for data pipelines.
- Reduce rework costs and accelerate time-to-value.
Use Case 2: GenAI Lifecycle Monitoring
Ensure your AI initiatives deliver business value:
- Validate training data quality and RAG knowledge sources.
- Monitor for hallucinations, bias, and performance drift.
- Track model operational metrics in real-time.
Use Case 3: Safe Self-Service Analytics
Empower your organization with confident data exploration:
- Embed real-time data health indicators in catalogs and BI tools.
- Monitor dataset usage patterns proactively.
- Build trust through transparency and validation.
The Actian Advantage: Five Differentiators That Matter
- No Data Sampling: 100% data coverage for comprehensive observability.
- No Cloud Cost Surge Guarantee: Predictable economics at scale.
- Secured Zero-Copy Architecture: Access metadata without costly data copies.
- Scalable AI Workloads: ML capabilities designed for enterprise scale.
- Native Apache Iceberg Support: Unparalleled observability for modern table formats.
Get Started
Take a product tour and better understand how to transform your data operations from reactive chaos to proactive control.
Subscribe to the Actian Blog
Subscribe to Actian’s blog to get data insights delivered right to you.
- Stay in the know – Get the latest in data analytics pushed directly to your inbox.
- Never miss a post – You’ll receive automatic email updates to let you know when new posts are live.
- It’s all up to you – Change your delivery preferences to suit your needs.