Databook: How Uber Turns Data into Exploitable Knowledge With Metadata
Actian Corporation
February 17, 2020
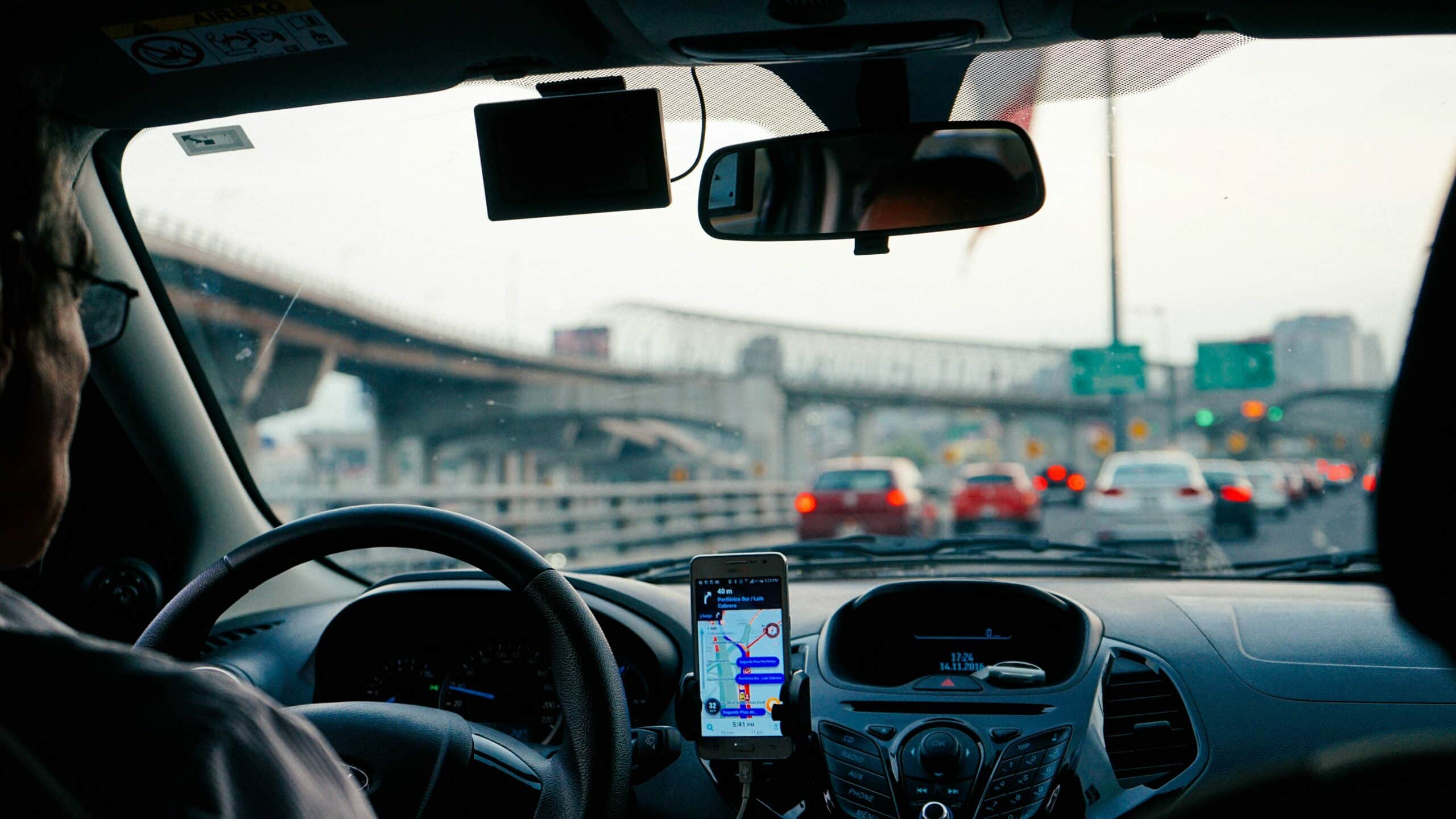
Uber is one of the most fascinating companies to emerge over the past decade. Founded in 2009, Uber grew to become one of the highest valued startup companies in the world. In fact, there is even a term for their success: “uberization” which refers to changing the market for a service by introducing a different way of buying or using it, especially using mobile technology.
From peer-to-peer ride services to restaurant orders, it is clear that Uber’s platform is data-driven. Data is the center of Uber’s global marketplace, creating better user experiences across their services for their customers, as well as empowering their employees to be more efficient at their jobs.
However, Big Data by itself wasn’t enough; the amount of data generated at Uber requires context to make business decisions. So like many other unicorn companies, such as Airbnb with Data Portal, Uber’s Engineering team built Databook. This internal platform aims to scan, collect, and aggregate metadata to see more clearly the location of data in Uber’s IS and their referents. In short, a platform that wants to transform raw data into contextualized data.
How Uber’s Business (and Data) Grew
Since 2016, Uber has added new lines of businesses to its platform, including Uber Eats and Jump Bikes. Some statistics on Uber include:
- 15 million trips a day.
- Over 75 million active riders.
- 18,000 employees since its creation in 2009.
As the firm grew, so did its data and metadata. To ensure that their data & analytics could keep up with their rapid pace of growth, they needed a more powerful system for discovering their relevant datasets. This led to the creation of Databook and its metadata curation.
The Coming of Databook
The Databook platform manages rich metadata about Uber’s datasets and enables employees across the company to explore, discover, and efficiently use their data. The platform also ensures their data’s context isn’t lost among the hundreds of thousands of people trying to analyse it. All in all, Databook’s metadata empowers all engineers, data scientists and IT teams to go from just visualizing their data to turning it into exploitable knowledge
Databook enables employees to leverage automated metadata in order to collect a wide variety of frequently refreshed metadata. It provides a wide variety of metadata from Hive, MySQL, Cassandra and other internal storage systems.To make them accessible and searchable, Databook offers its consumers a user interface with a Google search engine or its RESTful API.
Databook’s Architecture
Databook’s architecture is broken down into three parts: how the metadata is collected, stored, and how its data is surfaced.
Conceptually, the Databook architecture was designed to enable four key capabilities:
- Extensibility: New metadata, storage, and entities are easy to add.
- Accessibility: Services can access all metadata programmatically.
- Scalability: Support business user needs and technology novelty.
- Execution: Power & speed.
To go further on Databook’s architecture, please read their article https://eng.uber.com/databook/
What’s Next for Databook?
With Databook, metadata at Uber is now more useful than ever.
But they still hope to develop other functionalities such as the abilities to generate data insights with machine learning models and create advanced issue detection, prevention, and mitigation mechanisms.
Sources
Subscribe to the Actian Blog
Subscribe to Actian’s blog to get data insights delivered right to you.
- Stay in the know – Get the latest in data analytics pushed directly to your inbox.
- Never miss a post – You’ll receive automatic email updates to let you know when new posts are live.
- It’s all up to you – Change your delivery preferences to suit your needs.