Data Warehouse Best Practices
Teresa Wingfield
October 26, 2021
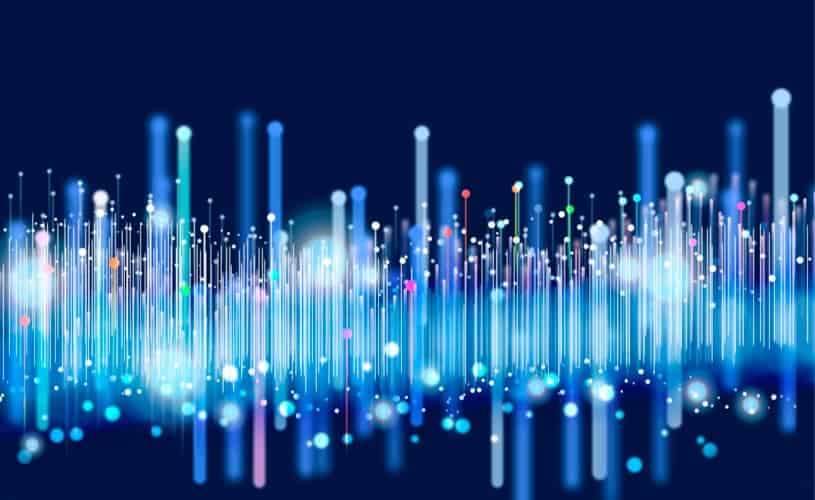
In every industry, the need to follow best practices exists. Data warehouse best practices are no exception. Best practices are methods or techniques accepted as a good way or best way to accomplish an activity, process, or practice. All practices evolve, but the best way to start is with a foundation of best practices and then adapt those practices to meet the specific needs of an organization. Organizations that continually evolve their best practices based on industry, customer, and internal feedback will create unique best practices resulting in a strategic, tactical, or operational advantage over a similar organization serving the same markets.
Best practices enable assets, capabilities, and resources to deliver value to the organization, stakeholders, and customers. A data warehouse can be a strategic resource for any organization. Developing a data warehouse practice into a unique capability requires making the data warehouse best meet the organizational objectives that the data warehouse technology supports.
Data Warehouse Best Practices
Data within an organization is sometimes not leveraged as much as it can be. Many organizations find themselves making decisions using best effort or expert opinions in most cases. These decisions can become more powerful and meaningful when backed with intelligent data, information, and knowledge relative to the needs of data consumers. To do this, organizations have to work as a team and remove as many silos as possible related to the services and products they deliver and support. Data exchanges between customers and all the functional units in the organization help make this happen.
Organizations rely on many best practices in various functions to perform as efficiently as possible. There are best practices for managing people, methods, processes, and technologies. Listed below are several best practices and data warehouse considerations that should be adopted within an organization to help enable value from a data warehouse:
- Identify what decisions need to be made within each functional unit of the organization and how data supports their conclusions. Data should have a purpose. Data collected that does not have a goal is a waste of the organization’s precious resources. The organization must be efficient and effective with data collection, including exchanging data between functional units, transforming data into information, and then shifting it into knowledge for decision support.
- Create models. Service models, product models, financial models, and process models help organizations understand data exchanges and the data needed by different stakeholders to define and architect the data warehouse data model. The data model helps the organization understand the value chains between different data consumers and how data should be presented.
- Understand decisions that need to be made by each consumer of the data in the data warehouse. Analyze and understand data needs for each consumer of the data.
- Decide governance, risk, and compliance (GRC) policies, processes, and procedures. Managing data is very important to any organization and should be treated with utmost care and responsibility. Data activities within the organization have to operate efficiently, effectively, and economically to avoid risk and resource waste.
- Decide the type of initial data warehouse. Decide whether a 1-, 2-or 3-tier architecture is the best initial approach for your data warehouse. Remember, data warehouses support analytical processing and are not suitable for transactional processing.
- Decide if the data warehouse should be on-premises, cloud or hybrid. This includes understanding the budget available for the overall initial program/project and its impact on the decision.
- Decide initial sources for input into a data warehouse. Remember, data sources can grow over time as the needs of the organization grow. It is essential to make sure adding new data sources is easy to accomplish.
- Create a project plan to break up the delivery approach into manageable units for showing value quickly using the data warehouse. Don’t try to be perfect with a never-ending project or try a big-bang approach. Show value as soon as possible. Be agile, get good enough and plan for continuous improvement.
- Decide data warehouse needs for availability, capacity, security, and continuity. The data warehouse has to be available when needed, have enough capacity to support demand, secure and maintain levels of confidentiality, integrity, and be available to those who need it. For continuity, the data warehouse should be included in business impact analysis and risk assessment planning. Usability and performance are also considerations for data warehouse warranties to its consumers.
- Decide how often data needs to be loaded and reconciled, based on timeliness and relevance of data change, from data warehouse sources for decisions. Use Extract, Transform and Load (ETL) to help migrate data between sources and destinations. Data warehouse staging is a best practice to help stage data on a regular schedule for data warehouse decision needs.
- Setup data for reporting, analytics, and business intelligence. Data warehouse reporting best practices have to be enabled for ease of use by data consumers. The consumer should be able to create dynamic reports with ease from the data warehouse quickly.
- Follow agile best practices for change, release, and deployment management to help reduce risks and increase knowledge transfer. These best practices should integrate and align with other best practices in the organization.
- Make sure to hire experienced people who are experts in data warehouse planning, design, and implementation. Bringing the right team together is one of the most important best practices for data warehouse design, development and implementation. No matter how good the technology is, the overall results will be disappointing without the right people. Project managers, business analysts, data analysts, data engineers, data architects, security analysts, and knowledge managers are key roles that can help with a successful data warehouse.
Best practices in business intelligence and data warehousing go hand in hand. The better the data warehouse technical infrastructure, the better the organization can collect, store, analyze, and present the data for consumer intelligence. Organizations have to be careful of insufficient data quality resulting in bad data for business intelligence. The data warehouse should easily support tools or applications that need the data for business intelligence. Reporting, data mining, process analysis, performance benchmarking, and analytical tools support business intelligence and should be quickly implemented without creating homegrown solutions for the data warehouse.
In Summary
This blog has discussed many data warehouse best practices. Depending on the organization and challenges they have experienced, more best practices can be added to the ones listed above. Best practices can come from anywhere in the organization based on experiences, challenges, and the overall market dynamics that the organization faces. Data warehouses and enterprise data hubs are fast becoming a strategic component for many organizations. Since a data warehouse is a large project that will mature over time, it should become a major program in the organization. Data is the blood that runs through the organization; this will not change. Data management will advance with emerging technologies, but the purpose will remain to help the organization make better informed and more timely decisions. Make a plan to start or improve your data warehouse outcomes by using best practices and selecting the right partners, technologies, and software to aid your journey.
Actian combines one of the industry’s fastest hybrid-cloud data warehouses with self-service data integration in the cloud to create better customer insights. With an easy, drag-and-drop interface, Actian Data Platform empowers anyone in the organization – from data scientists to citizen integrators – to easily combine, clean, and analyze customer data from any source, in any location.
Subscribe to the Actian Blog
Subscribe to Actian’s blog to get data insights delivered right to you.
- Stay in the know – Get the latest in data analytics pushed directly to your inbox
- Never miss a post – You’ll receive automatic email updates to let you know when new posts are live
- It’s all up to you – Change your delivery preferences to suit your needs